How to have a profitable digital service for data operation ?
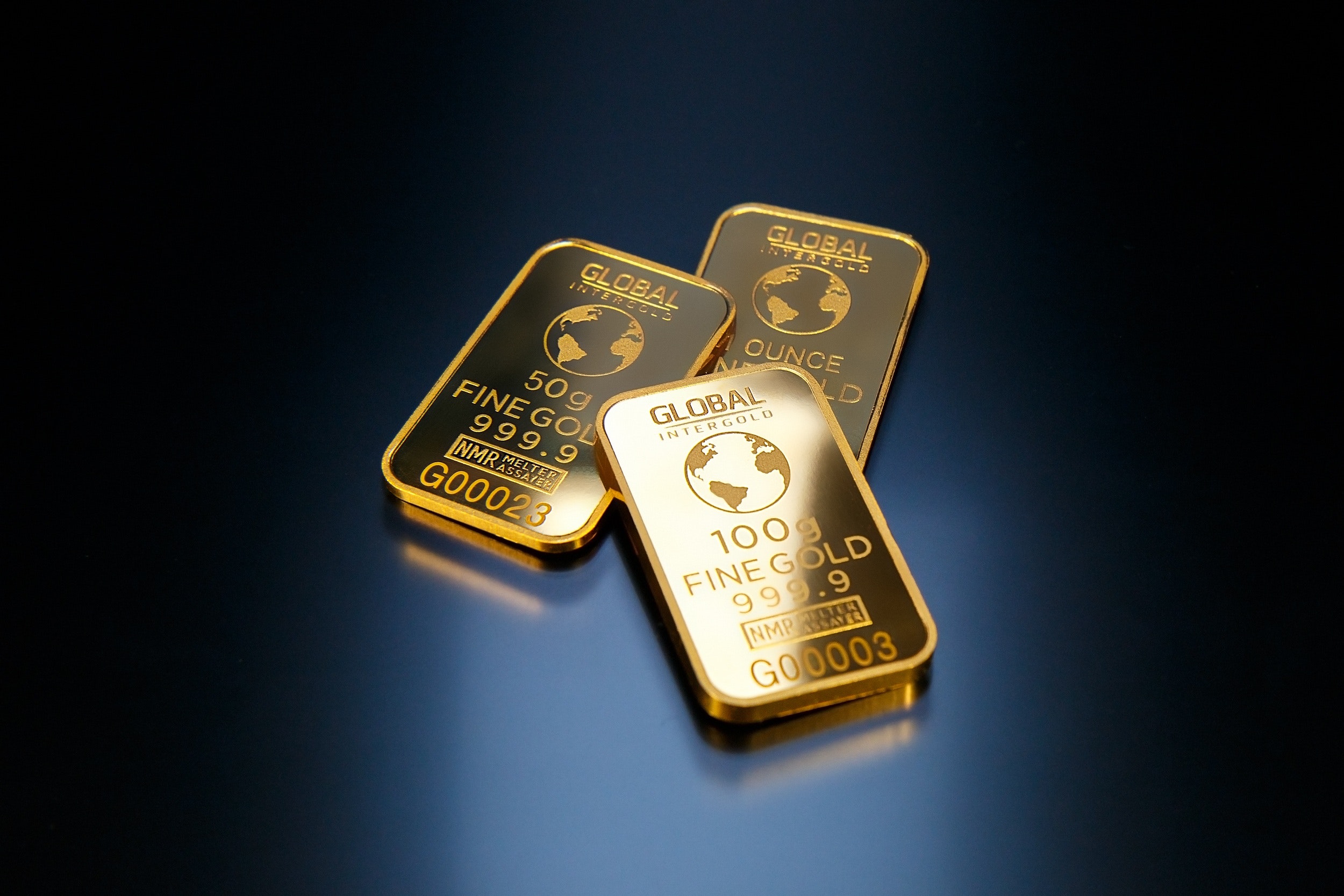
Digital transformation is a challenge for a company. Behind all the buzz words, there is a real need for change and the ability to look at the business in a new way.
This new way is based on data and how the creation or transformation of the information can create value. However, despite the increase of technologies that allow speed and power, most of the concepts used on the value creation are not new and have been either inspired or reused from other domains.
So, where is the digital value then ?
The value, and sometime the disruption, come from the ability to create a model of the reality that allows to apply a digital process on it and give the created value to the next process. Transferring data to the next digital actor is a major difference in the way information has to be considered.
Historically, information (considered as knowledge) has been considered as a valuable private (or secret) asset with a price related to its rarity. Whereas this is still the case for some of the information, a significant part of the value is also created by the ability to connect information together and to isolate new information by synthesis, correlation or trend analysis.
Even if that can sound scary, as sharing information with others implies to be in open competition with them, it is also allow the creation of value on information that the original system does not always own (or master).
To give an example, lets look at a service that allow to realize the best numbers of clothes from a large band of tissue.
This implies to build a model of the clothes pieces with constraints (like orientation of the pattern, deformation due to stretch …) and find the best process to arrange the layout to reduce the waste of material.
The understanding of fashion or use of the pieces does not matter, the output of the service is send to a physical equipment that will convert this data into real processing of the material

The heart of a digital service is the data … and we create more and more data every day.
To embrace the amplitude of the change, the interesting point is that we have created more data in 2017 than in the last 5000 years.
To face the need to store and connect those data, we have seen the emergence of concept of data lake which is a giant storage of all the data sources in a consistent location. However, even if we can store those data, this comes with an operational cost, and the question to create value from it is still unresolved.
Applying technologies from one domain to another is a clear source of high value; limiting the cost of maturity of the technology and allowing focus on the adaptation to the new domain.
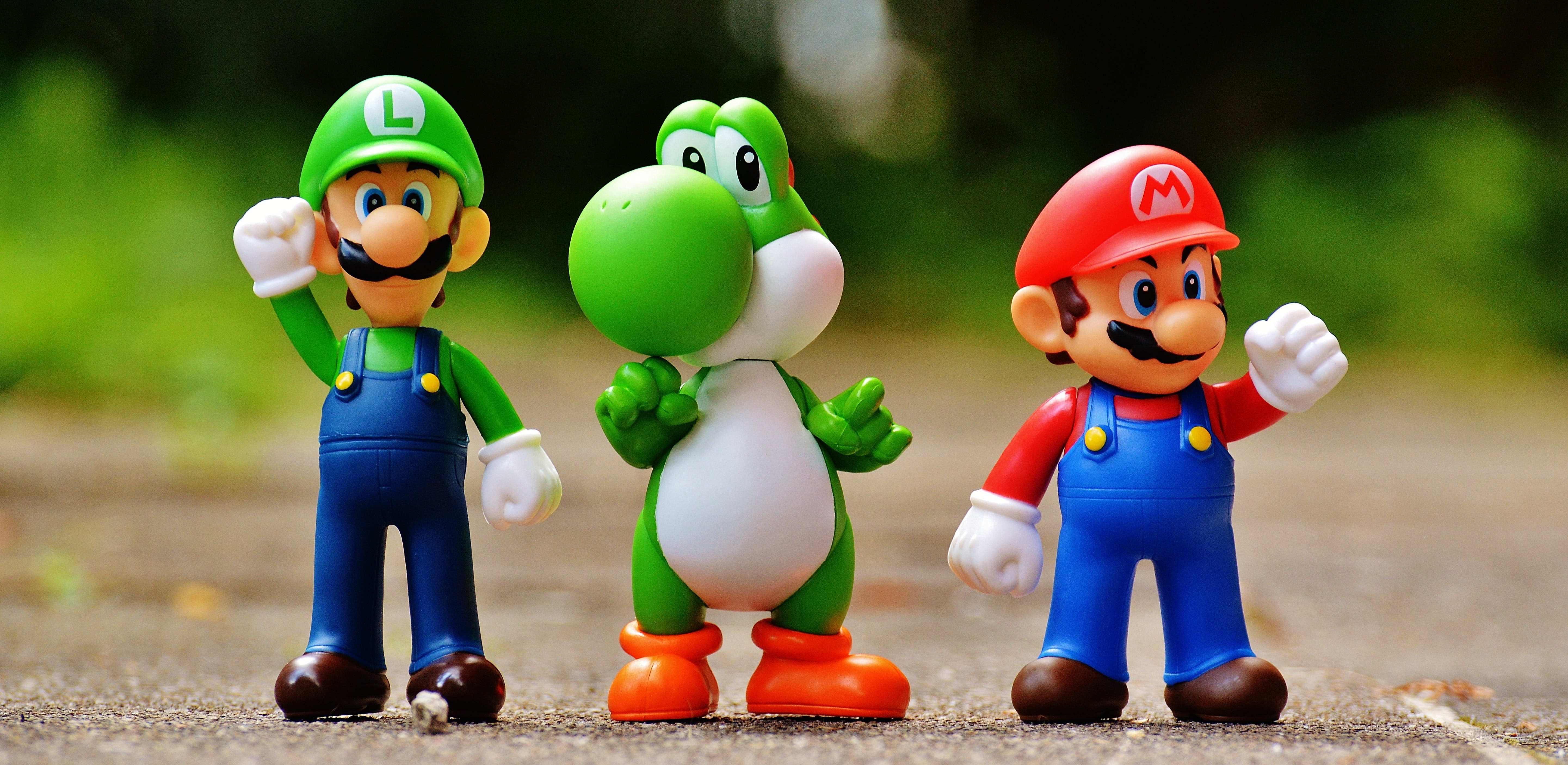
One example of a domain shift, is the creation of 3D images.
A few decades ago, the cost of the creation of those images was important and time consuming. The model used to create the image was based on the idea of defining color of each point from a ray of light thrown into the scene observed (ray tracing).
Whereas the model was good, for the basic rendering, it was reaching its limit for complex or detailed rendering like architecture.
One disrupting approach has been to reuse the model of heat propagation (thermodynamic) in the context of 3D view by replacing the heat by source of light.
The new model called radiosity is now applied for almost all 3D movies to create realistic scenes.
Coming back to our question, we need to wonder what are the models and processes that we can apply or combine from other domains in our industry to create new value or data.
The most important idea is to apply an existing concept to the avionic and passenger knowledge in a way that require few investments compared to the value added.
To illustrate this, we can look as the use case of the Pokemon Go game. Using a map, basic augmented reality, GPS or reward base game are not a disrupting innovation.
However, the innovation was to imagine a model where the smart combination of all creates a game that is appreciated as an healthy practice because it push users to exercise, capture funding from shops and facilities to bring customers close to them, and continue to collect money from users based on their addiction to a known brand.
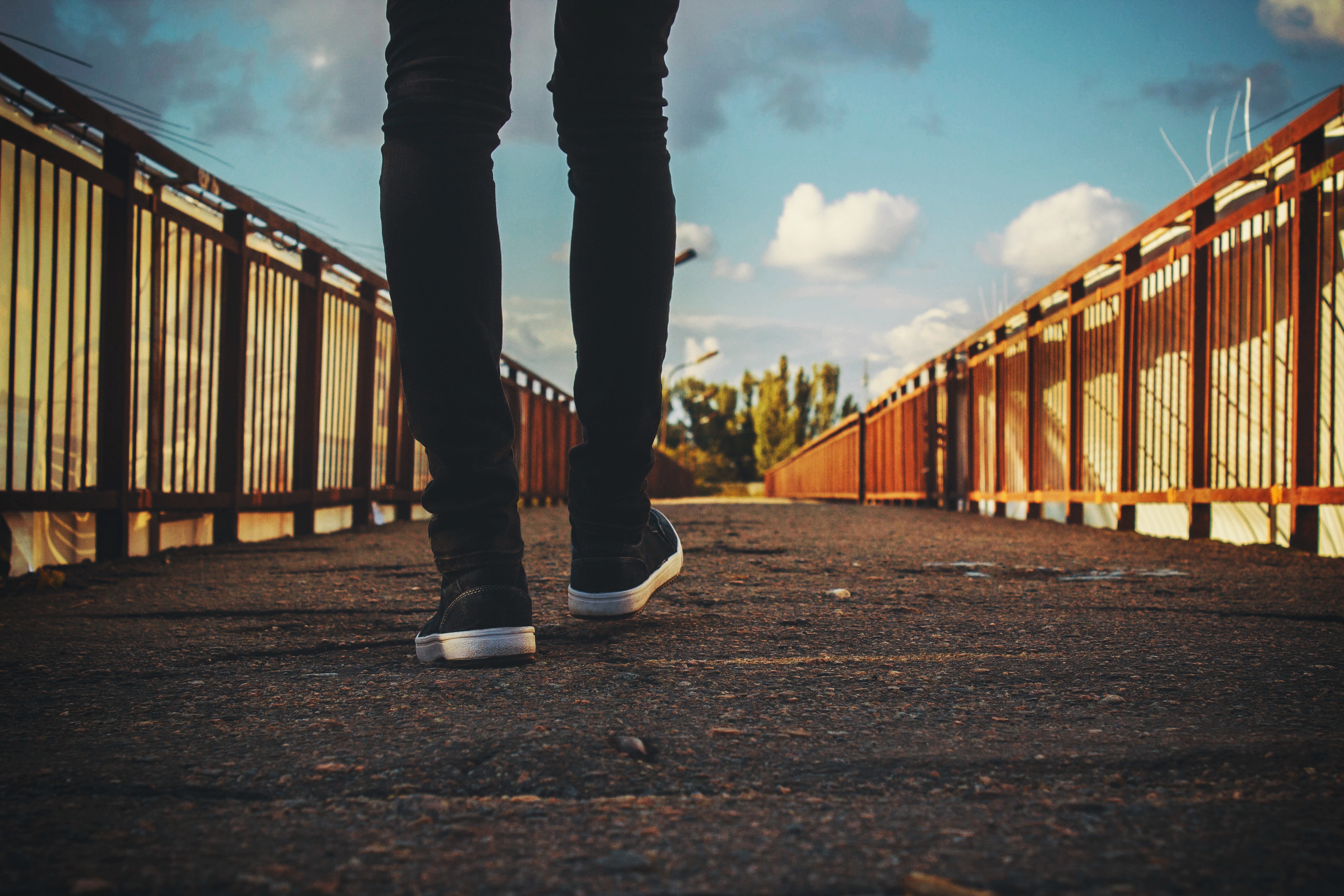
In this example, most of the technologies are not provided by the creators of the product but used by them.
The value is created by the association of each data set in a consistent environment.
In the avionic and in digital, the cost of the acquisition of the system is minor compared to the cost of operation of the system to fulfill service engagement.
Understand operation
Operation is a major source of data as the efficiency of activities relies on the ability to use, create or transfer quickly the information or the goods between users or system without interruption of the flow. As operation is a wide domain, we will dive in this talk into two aspects of it : data flow and scheduling.
Like the theory of strength of materials, to be able to improve operation, we need to analyze the system to understand the points that will be stressed by the increase of the speed and the element of our process that will generate frictions and slow down the service expansion or efficiency
For this, before looking at the potential value added, we need to isolate the singularities of our domain to create a model that enables value.
Data communication
Communication is not restricted to create links between actors.
Behind the size of the pipes, the ability to have the correct management of the flow and the right scheduling is a mandatory attribute.
To understand this first aspect, we can start with an image of our daily life : the water pipe of our house.
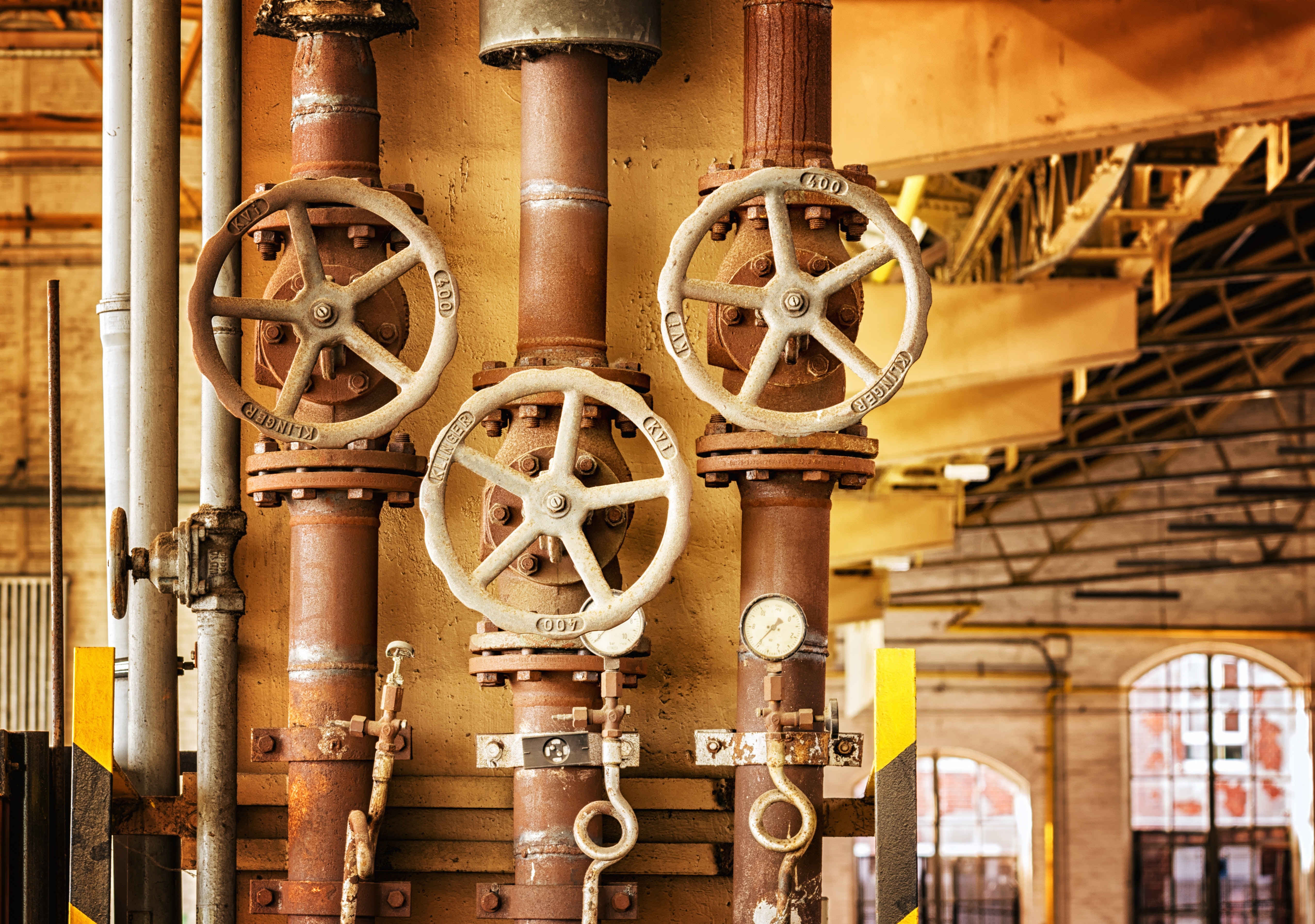
The flow of water that we can receive in every sink is related to the size of the previous pipe (coming from the street) and the way that the water is distributed.
In this description, the water is coming from the outside and distributed to the user; the other part to the model is the evacuation back to the street.
Both flows are almost symmetrical (for your own sake, you expect to have almost the same amount of water coming in and out of your house).
In our domain, the first difference is that, due to the medias, we consume more information that we produce (by the use of the system); this implies that the flow is mainly asymmetrical.
The second difference is that, most of the time, the same pipe is used for both directions (in and out) which implies that a good management of priorities and transit conflicts has to be defined.
Mobility
On top of this, the aircraft has an important singularity which is its mobility and availability. Despite most of the other objects of our life, an aircraft is moving fast from one location to another with a non persistent connection (and sometime even loose power).
This singularity implies that, even if similar models can be found in the industry, the combination of those criterii will prevent a direct simple reuse

If you compare a plane with a phone,and look at the mobility in term of geographical location, the aircraft will have an extensive use of multiple infrastructures geo-dispersed.
It will move in a day from one region of the world to another which implies to change from one operator to another.
The second aspect of the mobility is the fact that the aircraft will change also the type of connection used to transfer the data (4G, wifi, SATCOM …).
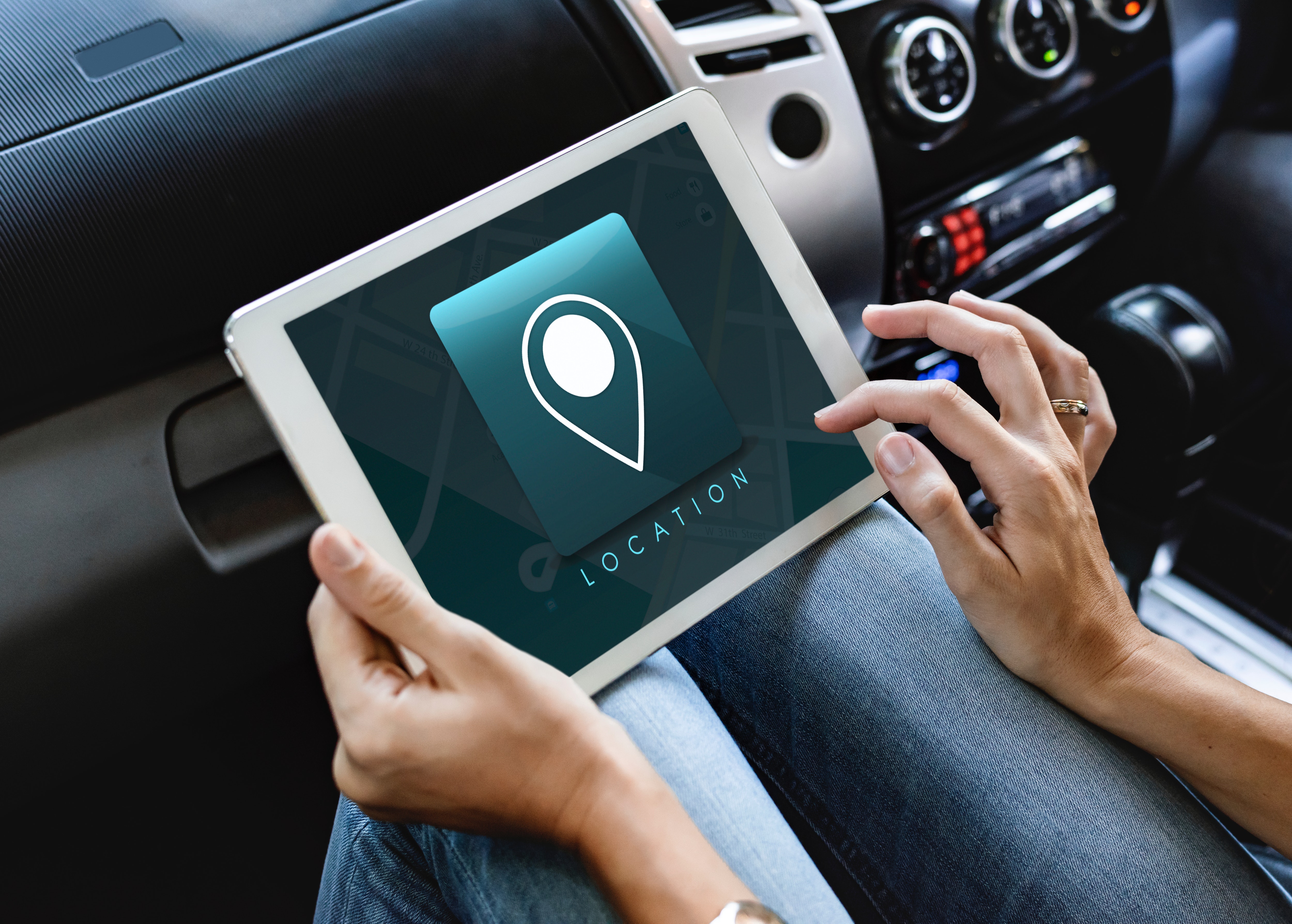
The model of connection of our cellular phone will expect a hand over between beans and have a cost model based on a 4G or WiFi only; its definition can be partially applied but will not provide by default an efficient way to select the right channel and to do the hand-over between the pipes.
Flexible data flow
To analyze a practical use case of the data flow, we can look at the example of a transcontinental flight from LAX to CDG.
- The aircraft will receive at the airport (through Wifi/4G) information coming from a local network of an american provider
- After take off, the aircraft will use KA SATCOM to send information to the data-center of Inmarsat in New York
- When it will reach the middle of the ocean, the flow will be transferred to the data-center in Amsterdam
- Landing in CDG, the aircraft will connect to the local infrastructure of the airport that will probably involve an European provider
One challenging aspect of the use of multiple pipes and the geo-dispersion is that the system will need to have the ability to limit the data re-transmitted after interruption.
Data efficiency has an important financial ability on the solution and on the viability of any connected service
Availibility
If we observe now the intermittent connection of the aircraft to the network, we can isolate another additional singularity :
Most of the connected equipment expect to be powered and always connected to be able to perform maintenance and preparation for operation on a different schedule that the operation itself.
For example, a media center will create picture thumbnails and data indexation in the background when the user is not fully using the device
Identity
Finally, the aircraft needs to combine in its data, three type of identities :
- its identity (airline, tail, flight)
- the identity of its users (passenger, crew, maintenance …)
- the identity of the services (VOD, VOIP, health …)
Unlike a car or a media box, the users of the system are changing almost on every flights which implies to reacquire the personalization data before and after each flight but also to transfer from owner to owner (pilot and crew) the history and characteristics of the aircraft.
Dataflow
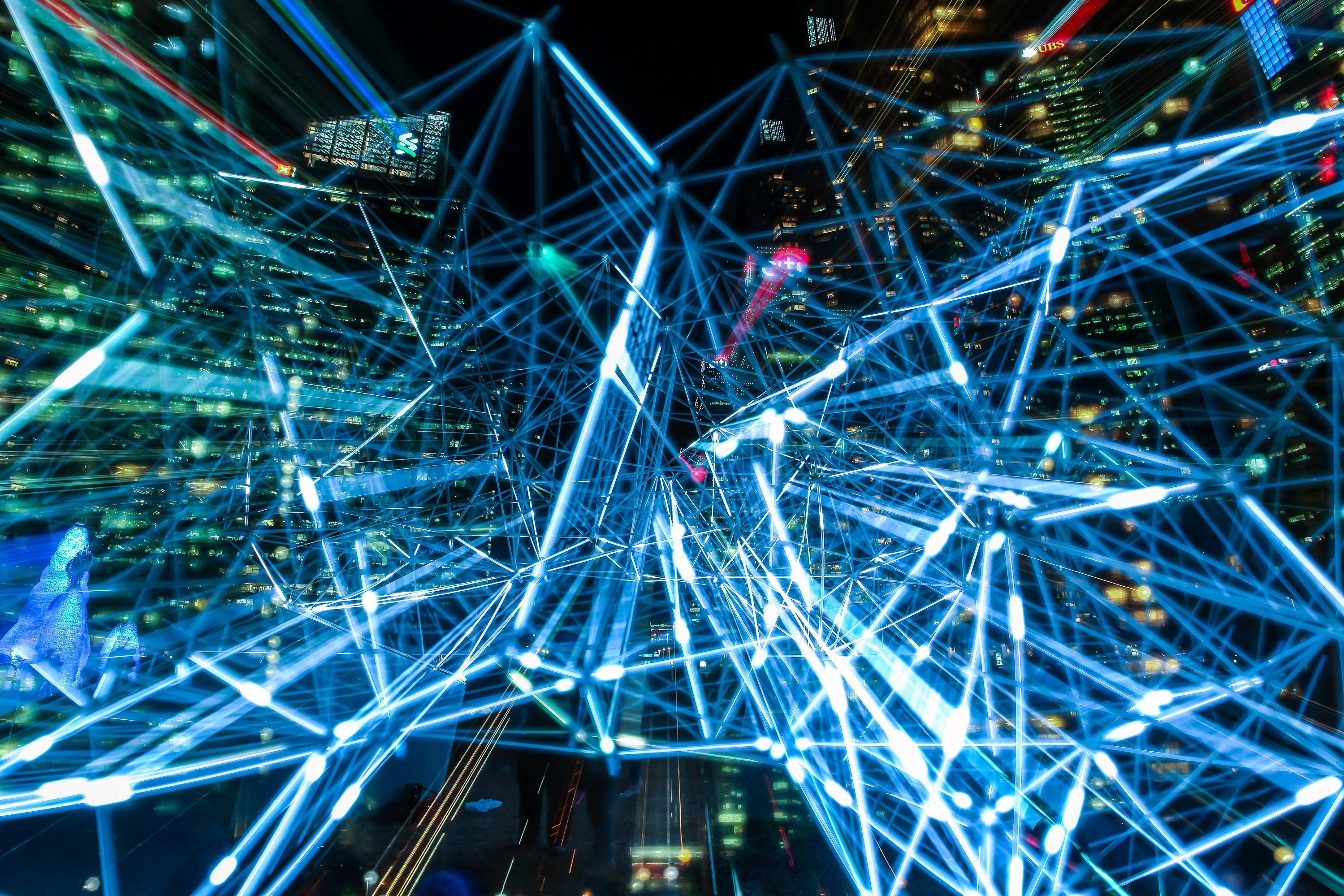

To move forward, in the modelization of operation, we need to analyze the type of data that the system has to process and the time information available.
Physical drivers
If we observe the schedule aspect of the passenger on the aircraft, we are in a transport model heavility based on a pre-planned activity which mean that the aircraft route and the passenger route is knowed in advance.
This model is close to long range rail (as short range rail preplanned only the volume of customers, not the individual path), however if we compare models, rail have the ability to load/unload passengers on the route which rarely apply to avionics.
On the other hand, the avionics model needs to provide content to the passenger which is not for the moment proposed in transport use case.
The schedule will be separated into two flows :
- one related to the aircraft
- the second one, related to the passenger.
Both will have to be consolidated to be able to optimize the use of the infrastructure.
If you analyze the aircraft flow, the organisation of the operation is very similar to the operation of a fleet of trucks with mobility, velocity and capacity.
Unless, we plan to use aircraft to deliver data to another location (which is unlikely to happen for now due to the cost), the velocity of the aircraft is only used in maintenance operation to predict the time of the arrival.
Data driver
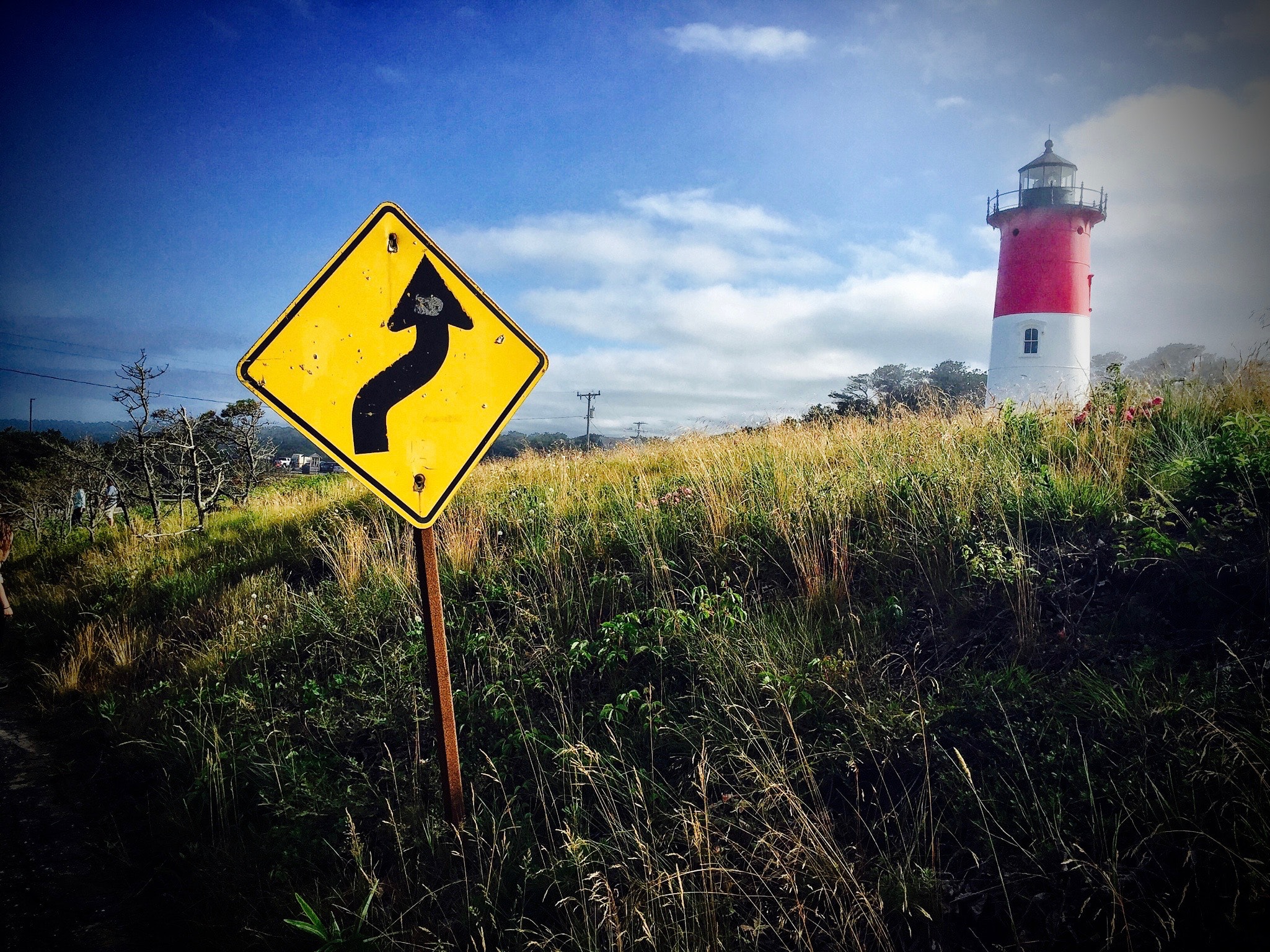
The second driver is that the data is not always from a point A to a point B but will follow different flow models :
- The data from operation will mainly come out of the aircraft (logs, tracking, messages)
- The data for passenger will mainly follow the passenger (in before flight, out after)
- The data for operation (media, information) will mainly come to the aircraft but based on the best interest for the group of passengers (and maybe on second priority on the interest of one single passenger)

To understand the benefit of cost optimization, we can have a quick simple analogy with the use of internet at home.
Assuming that 3 persons at home want to watch a movie after work. As each stream will need 4 Mb/s, you will need to provision a 12 Mb/s contract with a provider.
However, if you can move to a preplanned model of this activity, assuming that you have only 4 hours of streaming in a normal day, you should need only 12*4h / 24h capability which is 2 Mb/s.
This talk will focus on the value created by optimizing the operational cost of the data flow through a business model. It will target a cost optimization by reducing the infrastructure need and improving the delivery speed (by flow optimization)
This difference create an important value as the cost of an infrastructure is not linear compare to the size of the flow that is needed to support.
On an aircraft, assuming a normal operation of 3 flights a day with an average duration of 4 hours, the average availability will be :
- 6h on ground : 4 * 1.5h for low cost transmission
- 12h on air : 4 * 3h for high cost transmission
For the moment, ground transmission are heavility cheaper than the air transmission.
Unfortunately, unlike transport optimization of passenger, the data cannot be optimized by adjustment of the schedule of the flight.
This will be unlikely the case soon as the cost of fuel and the difficulty to schedule the traffic on airports is at a difference value scale than the transmission cost
This mean that the data optimization has to be realized first based on the flight activity and then on a classic IT operation (with technologies like SDN – Software Defined Network)
Manage group of aircrafts
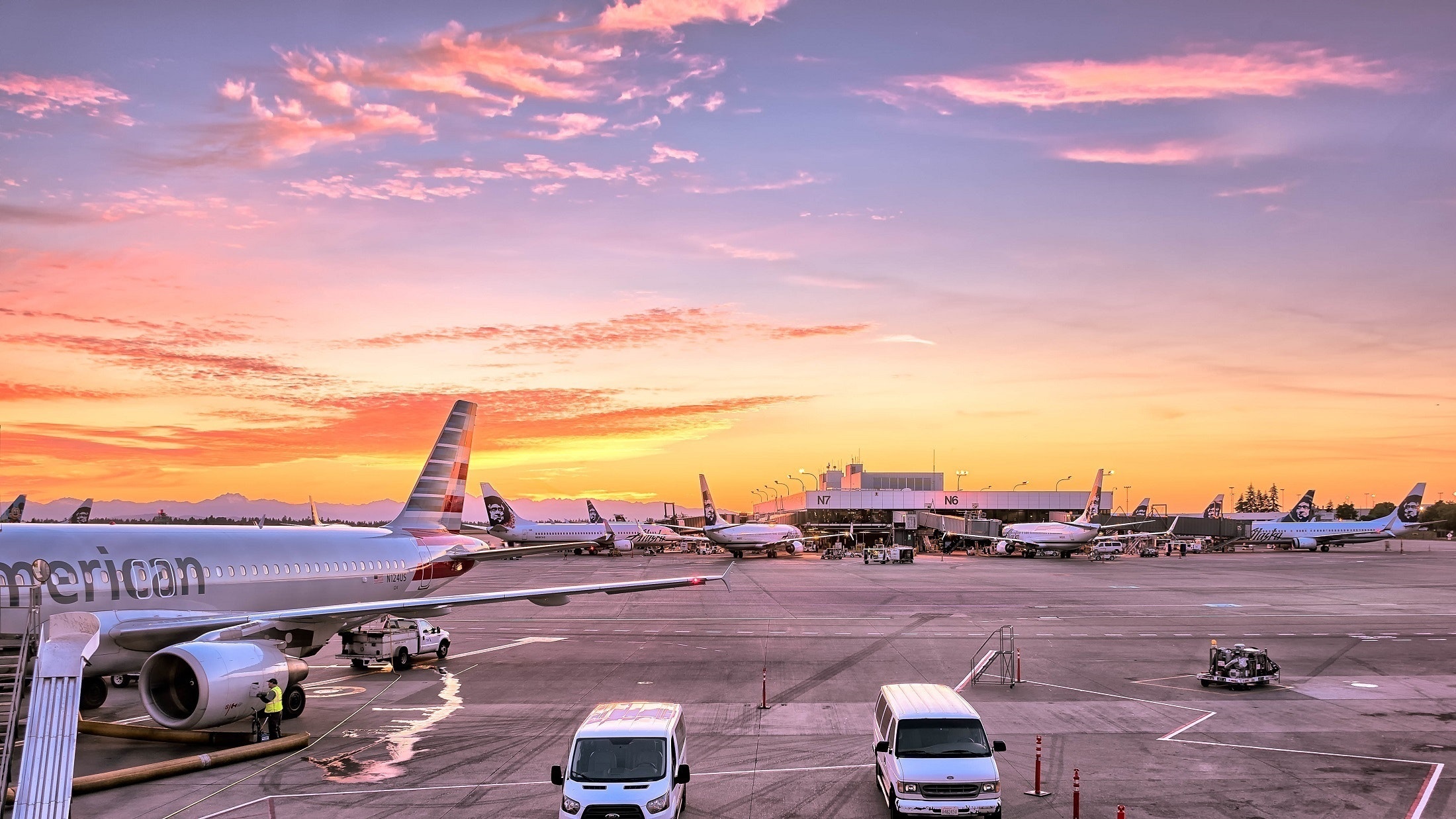
If we look now at the flight activity for data optimization, we can isolate some dimensions.
First of all, at the scale of a fleet, the cost of transmission can be reduced if some information are broadcasted for a portion of the transmission instead of always having transmission points to points.
A classic use case of broadcast is multimedia streaming of information like radio or television.
However broadcasting of data can only be used if the data is the same for all aircraft (like content) and if aircraft are using the same pipe at the same time (like SATCOM)
Impact of the aircraft operation
On the ground then, the data transformation will be affected by 2 main constraints : the availability of the power of the aircraft and the density of aircraft in the airport.
For the first one, the average procedure of maintenance will imply a reboot of the system every 24 hours minimum (and for some companies every 2-3 flights) as the avionic equipment diagnostics are only performed during the start of the system (POST).
Due to the power source (fuel), the aircraft are only powered when they are closed to their flight activity or when they are at the gate (secondary power supply) which imply a small availability of the system out of its main operation phase (like IFE)
Airport density
For the airport density, the quality of the wireless (or cellular) transmission is affected by the density of other devices or aircrafts.
For cellular, the aircraft use the local coverage of the airport and is affected by all others public devices present in the area (even if a professional contract can give priority to its communication)
For WiFi, as the network is provided by the airport, the bandwidth is shared between airlines and aircraft and sometime with the own need of the airport.
Assuming that each aircraft receive the same bandwidth, from the point of view of a single customer, the total size of transmission is by default proportional to the number of aircrafts at the airport.
So, as a consequence, an airline with a few aircrafts will receive an average total of information lower than another airline with a bigger fleet.
Time critical
Time criticality can be addressed at two levels :
- Architectural level
- Priority level
At the architecture level, a standard pattern called lambda architecture separate the collection of the data than need speed to the data than are processing by batch. From there, the data is consolidated in a serving layer and recombine for synthesis if needed
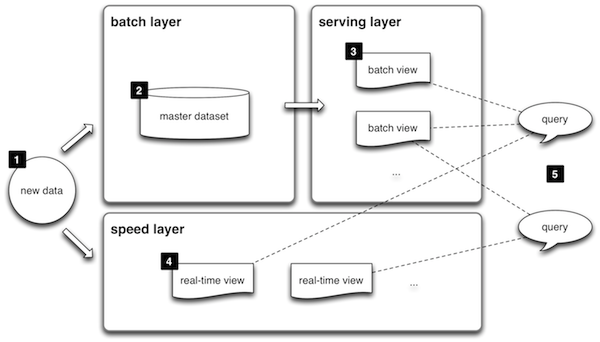
For priority, we need to look first at the type of flight, long flight and short flight do not have the same profile : a long flight will have fewer availability period on the ground but longer ones due to the operations like refuel.
This mean that if 2 aircrafts arrived at the same time, a long flight should have the priority for time bounded data as its later availibility is not guaranteed.

But what is the influence of time bounded activity ? On a basis approach, time bounded activities (like delivery of payment logs) can be approached by initial priority assignment : a security log is more important than an health log.
However, most of the time, a dynamic adjustment is needed to take into account constraints like “Last available slot before deadline” or “on demand request for analysis”.
Non linear
The final parameter to take into account is the non linear workload created by recurrent needs.
For example, if a transfer of 2 Mb/s is needed every 10 mn, and another transfer of 5 Mb/s is needed every hour, without appropriate schedule, a spike of 7 Mb/s will be observed every hour, whereas a simple offset of the 5 mn will only create a spike of 5 mn.
This example, that will be refined in a further tech talk, at the scale of all various services requesting recurrent data, highlights huge potential of optimization.
Find the value
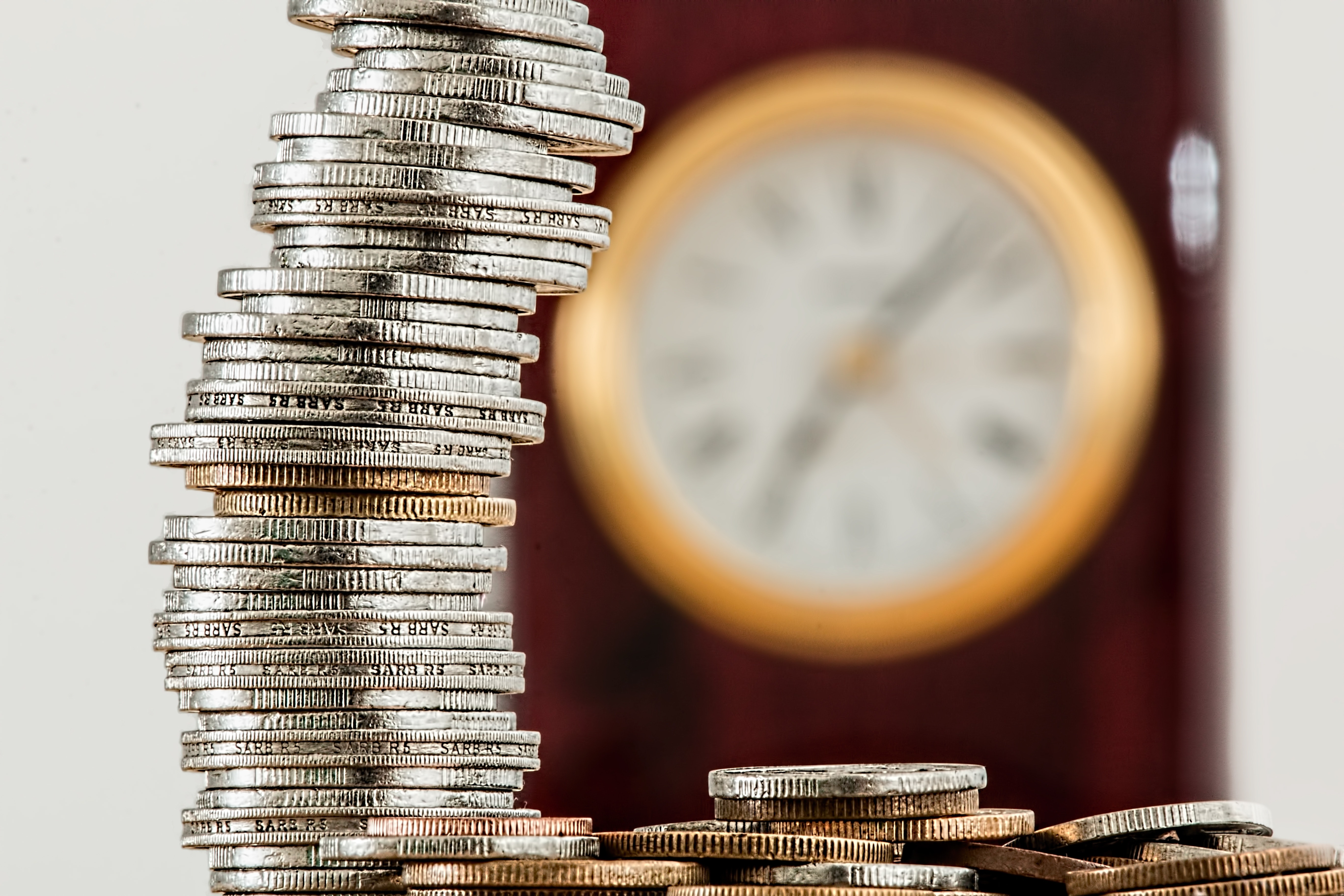
The value of those optimizations increase if the cost of the infrastructure to transmit is a dimensionning factor.
As the data flow is increasing at a higher factor than the increase of the infrastructure, the optimization of the communication has a growing value
In summary, the optimisation of the data transmission brings a direct valuable cost saving on the infrastructure and cost of transmissions, but needs, to be efficient, to take into account several dimensions :
- Aircraft activity
- Airport activity
- Transmission to provider activity
This is a multi-dimensional optimization that needs (thanks to a model) to take benefit from the pre-planning and realtime smart adjustment.
For example, the system has to decide if the update of a the content before a long flight is more important than gathering the user behavior data of the previous flight; or if a data analysis is more important than the connecting information for the passenger.
The result is an optimization of the direct value : the resource usage (like fuel) and an increase of the average speed of availability of the information (indirect value)
As we see, the digital value is found on data operation by adopting proven model of flow optimization found in transport or delivery.
The differentiation is created by defining the valuable information that will be used to aggregate existing models and adapting it to the avionic eco-system.
Building partnership with the owner of those models, is a good win-win situation as it provide additional use cases for the owner and create an additional value in the eco-system.
For example, the final optimized model for maintenance will probably aggregate a stock model to ship and provide spare on time, a tracking system for local team optimization and a schedule optimization tool customized for avionic life-cycle.
